Doug Kerr
Well-known member
Bart van der Wolf recently performed what we might call a "simulation" of mitigating the effects of diffraction through the use of deconvolution. He posted an excellent report on his procedure, with links to the test images, in Luminous Landscape in July. (In fact only the diffraction is "simulated" - its mitigation is the real thing.)
I think the results are so important that I wanted them to be reported here. Bart is pretty busy looking after our interests in Europe, so I volunteered to give a synopsis here. Hopefully Bart will be able to pile on and fix anything I got wrong.
Bart started with a shot of a building face with many interesting areas and surfaces for photographic testing.
Then, working in a program used for astrophotography, he simulated the effects of diffraction, as would have been caused by an aperture of f/32, on the image. He did this by convolving the original image with a "PSF kernel" - a digital description of the diffraction blur figure (Airy disc). Well, actually, part of one (a square piece of it 9 x 9 pixels - that was as big a map as the software could handle).
Then, he took that "afflicted" image and attempted to "back out" the effects of the diffraction by deconvolving that image with the piece of the PSF kernel.
As to the result, you can judge that from this display of the image in its three stages:
In the center (1), we have a small piece of the original image (at original resolution - nothing here has been downsampled). To its left (2), we see the same piece of the image that had been afflicted by the simulated f/32 diffraction. To its right (3), we see the final result of the effort to mitigate the impact of the diffraction.
The final result looks pretty well "healed" to me - and then some.
I'm very excited about he implications of this "simulation".
If you want to read Bart's original report on the LL forum, here is the link (the report is at reply no. 66 - I don't know how to link to that directly):
http://www.luminous-landscape.com/forum/index.php?topic=45038.60
The three images, in 16-bit form and original resolution, can be accessed from there, along with a table showing the 9x9 matrix describing the diffraction PSF.
We owe Bart much gratitude for providing this clear and powerful demonstration of the potential of what may be a very valuable image enhancement technique.
Best regards,
Doug
I think the results are so important that I wanted them to be reported here. Bart is pretty busy looking after our interests in Europe, so I volunteered to give a synopsis here. Hopefully Bart will be able to pile on and fix anything I got wrong.
Bart started with a shot of a building face with many interesting areas and surfaces for photographic testing.
Then, working in a program used for astrophotography, he simulated the effects of diffraction, as would have been caused by an aperture of f/32, on the image. He did this by convolving the original image with a "PSF kernel" - a digital description of the diffraction blur figure (Airy disc). Well, actually, part of one (a square piece of it 9 x 9 pixels - that was as big a map as the software could handle).
Then, he took that "afflicted" image and attempted to "back out" the effects of the diffraction by deconvolving that image with the piece of the PSF kernel.
As to the result, you can judge that from this display of the image in its three stages:
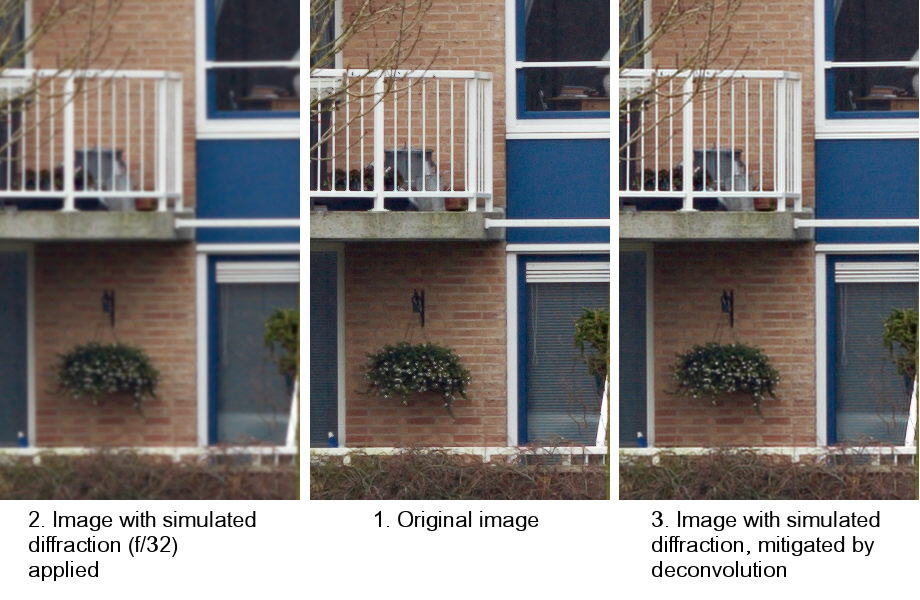
In the center (1), we have a small piece of the original image (at original resolution - nothing here has been downsampled). To its left (2), we see the same piece of the image that had been afflicted by the simulated f/32 diffraction. To its right (3), we see the final result of the effort to mitigate the impact of the diffraction.
The final result looks pretty well "healed" to me - and then some.
I'm very excited about he implications of this "simulation".
If you want to read Bart's original report on the LL forum, here is the link (the report is at reply no. 66 - I don't know how to link to that directly):
http://www.luminous-landscape.com/forum/index.php?topic=45038.60
The three images, in 16-bit form and original resolution, can be accessed from there, along with a table showing the 9x9 matrix describing the diffraction PSF.
We owe Bart much gratitude for providing this clear and powerful demonstration of the potential of what may be a very valuable image enhancement technique.
Best regards,
Doug