Asher Kelman
OPF Owner/Editor-in-Chief
We accept use of filters, lenses with "character and post processing from Ansel Adams scraping and scratching negatives or drawing on them to "content aware clean up in Photoshop and other competing software.
So now we face an even more remarkable tool about to come top Adobe. The new software will allow simply sketching over an image and have the software steal the real texture of the underlying reference picture and build a realistic new object.
This work is a collaboration between Adobe and a UC Berekely researcher, or rather Adobe out hunting for new tricks of the trade.
Abstract
Realistic image manipulation is challenging because it requires modifying the image appearance in a user-controlled way, while preserving the realism of the result. Unless the user has considerable artistic skill, it is easy to "fall off" the manifold of natural images while editing. In this paper, we propose to learn the natural image manifold directly from data using a generative adversarial neural network. We then define a class of image editing operations, and constrain their output to lie on that learned manifold at all times. The model automatically adjusts the output keeping all edits as realistic as possible. All our manipulations are expressed in terms of constrained optimization and are applied in near-real time. We evaluate our algorithm on the task of realistic photo manipulation of shape and color. The presented method can further be used for changing one image to look like the other, as well as generating novel imagery from scratch based on user's scribbles.
Read the DPPreview.com introduction here and the actual source article here.
Asher
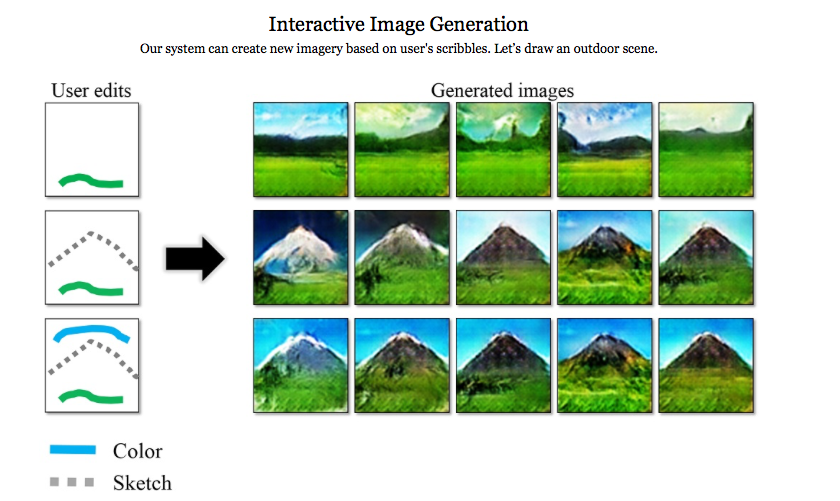
So now we face an even more remarkable tool about to come top Adobe. The new software will allow simply sketching over an image and have the software steal the real texture of the underlying reference picture and build a realistic new object.
This work is a collaboration between Adobe and a UC Berekely researcher, or rather Adobe out hunting for new tricks of the trade.
Abstract
Realistic image manipulation is challenging because it requires modifying the image appearance in a user-controlled way, while preserving the realism of the result. Unless the user has considerable artistic skill, it is easy to "fall off" the manifold of natural images while editing. In this paper, we propose to learn the natural image manifold directly from data using a generative adversarial neural network. We then define a class of image editing operations, and constrain their output to lie on that learned manifold at all times. The model automatically adjusts the output keeping all edits as realistic as possible. All our manipulations are expressed in terms of constrained optimization and are applied in near-real time. We evaluate our algorithm on the task of realistic photo manipulation of shape and color. The presented method can further be used for changing one image to look like the other, as well as generating novel imagery from scratch based on user's scribbles.
Read the DPPreview.com introduction here and the actual source article here.
Asher